STAN
RO3 : Parametric, stochastic and optimisation problems
Activities
This research operation focuses on the development of original methods for the construction, at lower cost, of solutions to problems that are beyond the reach of industrial calculation codes. The targets are problems with a very large number of degrees of freedom, non-linear, with the presence of several scales or interactions between several physics, or the desire to take into account uncertainties or parameter variations.
A first aspect of the activities concerns model reduction techniques, which are currently experiencing a real craze in the Mechanical Engineering and Applied Mathematics communities, as they offer a strong potential for developing innovative tools for high performance computing. In this context, OR is carrying out upstream work on the development of the LATIN-PGD (LArge Time INcrement Method - Proper Generalized Decomposition) computational strategy, introduced more than 30 years ago for the treatment of non-linear problems. PGD is now widely spread and developed in the international scientific community. The core of the activities around this method is now based on the construction of reduced models called "Virtual Abacuses". These parameterised abacuses, calculated offline, can be reused online in real time, allowing a wide range of totally new applications, for example in design/optimisation or for steering. The specificity of the work of the RO from an international point of view is the treatment of highly non-linear problems. Many results have been directly transferred to industry with collaborations with Airbus D&S, SAFRAN, CEA, SIEMENS... From an academic point of view, the RO is very involved in the model reduction community through the CNRS AMORE GdR which federates model reduction activities in France.
A second aspect of the activities is the response to the needs of industry for robust and efficient models and numerical simulation tools to assist in the design and optimisation of complex structures subject to environmental and production uncertainties. Amongst the many obstacles in the field of optimisation, the time required for simulation is a major bottleneck. One way to reduce the computational cost is to use surrogate models. The surrogate models developed within the OR are based on a non-physics-based approximation involving a Gaussian process regression of the quantities of interest generated from the original model and on physics-based model reduction techniques, such as the PGD already mentioned. Based on these two approximations, the RB exploits multi-fidelity techniques (also called variable fidelity, variable complexity) that combine these different models into a model hierarchy.
Manager
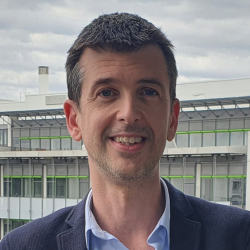
Equipe STAN
David NERON
Responsable de l'opération de recherche Problèmes paramétrés, stochastiques et optimisation
Professeur des universités