Equipe STAN
RO2 : Physics-based, data-enhanced, reality-integrated simulation
Activities
This research operation addresses the general issue of coupling between numerical simulation tools and available experimental information (test-calculation dialogue). It naturally encompasses the long-standing activities on the identification/recalibration of model parameters from measurements, by solving inverse problems, and on model validation, which remains a major industrial challenge for decision making. In this context, it deals with fertile themes such as the choice of a relevant regularisation, with, for example, the filtering of the experimental information to be retained among a flow of data (which can be large, heterogeneous, multi-scale, etc.), or the question of the management, propagation and quantification of uncertainties, resulting from measurements and model input data. It is therefore perfectly in line with the Predictive Science vision, which analyses all the factors likely to affect the reliability of digital simulations, by integrating modelling and partial knowledge of reality.
A key theme is also the hybridisation between models and data (hybrid twin concept), which aims to enrich an incomplete model with the information contained in the data. It goes beyond the recalibration of models, the idea being to compensate for the lack of knowledge of these models (unrepresented physics, unforeseen phenomena, variability, etc.) in order to be ever more faithful to reality. The contribution of learning techniques and artificial intelligence, constrained by physical considerations, is particularly studied here. The objective is to generate all or part of a model, or to correct the bias of a given physical model, from an appropriate analysis of a set of experimental observations.
Finally, the activities are oriented towards the sequential assimilation of experimental data and the dynamic control of physical systems, in connection with the recent DDDAS (Dynamic Data Driven Applications Systems) concept, which proposes a complete synergy between a physical system and its digital twin (digital servoing) to achieve a given objective. In this context, various approaches and tools (model reduction, adaptive multi-fidelity modelling, Kalman filtering, etc.) are used in addition to traditional techniques in order to obtain a simulation complexity that is compatible with the real-time constraint, while ensuring performance (just-in-time calculation at the right cost). The theme of optimal control is also addressed, in order to conduct a complete feedback loop with the physical system.
Manager
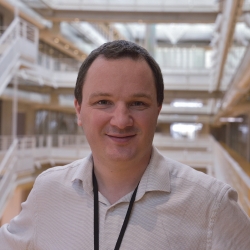
Equipe STAN
Ludovic CHAMOIN
University Professor
Head of the STAN team, Head of the research operation Simulation based on physics augmented by data and integrated with reality
Junior member of the IUF (Institut Universitaire de France)