Blending Physics-Based numerical simulations and seismic databases using Generative Adversarial Networks (GAN) for earthquake engineering and structural health monitoring
A new strategy to blend the outcome of physics-based numerical simulations with massive seismic databases is proposed, in order to improve earthquake simulation and structural health monitoring.
The approach relies on a set of adversarial learning techniques with a threefold purpose: (1) finding a reduced-dimensional non-linear representation of both synthetic and experimental data; (2) training a stochastic generator of fake experimental responses conditioned by the physics-based simulation results; (3) classify the damage class based on the structural response and predict the damaged response.
This methodology is applied to earthquake ground motion prediction and structural damage assessment. Regional three-dimensional high-fidelity numerical models accounting for both extended sources and complex geology are still limited to a low-frequency range.
Moreover, they are prone to significant uncertainties induced by a lack of data on small scale geological structures and rupture processes. Databases of broadband seismic signals recorded worldwide at seismological networks are used to retrieve some pieces of information on these small-scale data to generate realistic broadband signals from synthetic ones.
On the other hand, given the structural response of an undamaged structure, engineers need to predict the eventual damage beforehand, based on a monitoring network. The proposed tool demonstrates outstanding performances in encoding seismic signals, together with efficient generation capabilities and cluster capabilities, provided that the physics-based results carry enough information to properly condition the stochastic generator and classify the damage state.
In addition, the proposed method, fed only with raw data from both databases and numerical models, outperforms other random signal generators based on pre-existing expertise such as prescribed spectra and more or less complex phenomenological models.
Touhami, S.; Gatti, F.; Lopez-Caballero, F.; Cottereau, R.; de Abreu Corrêa, L.; Aubry, L.; Clouteau, D. SEM3D: A 3D High-Fidelity Numerical Earthquake Simulator for Broadband (0–10 Hz) Seismic Response Prediction at a Regional Scale. Geosciences 2022, 12 (3). https://doi.org/10.3390/geosciences12030112.
Young SECED Earthquake risk modelling Recent research advances from France and UK
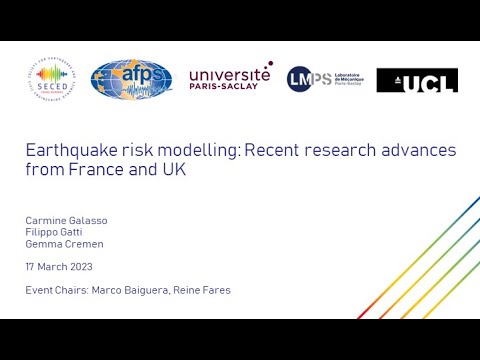
About the speaker
Dr. Gatti is assistant professor in Mechanics at CentraleSupélec, since 2019. He holds a joint PhD in Civil Engineering from Université Paris-Saclay and from Politecnico di Milano (2017) and a MSc (2014) and a BEng (2011) from Politecnico di Milano.
He is currently head of the research operation ‘Hybrid twins: simulation, learning’ within the Laboratoire de Mécanique Paris-Saclay and head of the master 1 program in Geomechanics and constructions. His research focuses on computational earthquake engineering and machine learning applied to civil engineering.